How Leveraging Machine Learning in Product Analytics Improves Insights and Actionability
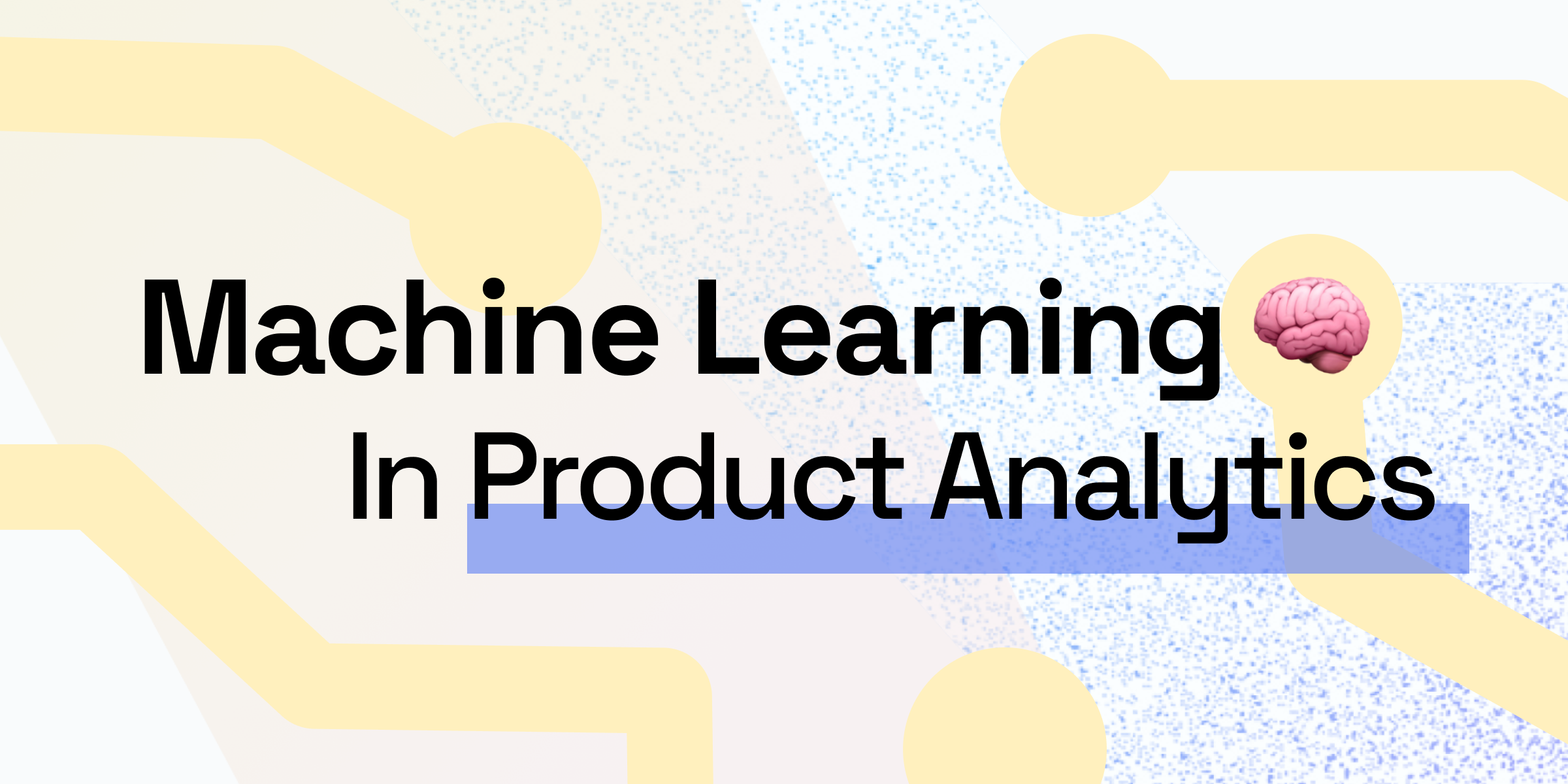
Product analytics traditionally hinged on examining user interactions to extract actionable insights. The integration of machine learning (ML) has elevated this process, enriching our understanding and our ability to predict future trends. Let's unfold how ML integrates into product analytics and the transformative advantages it introduces.
Beyond simple behavior-based categorizations, ML algorithms like K-means, DBSCAN, and Hierarchical clustering analyze multi-dimensional data. They can discern nuanced patterns in user behavior, resulting in richer, more diverse segments. This detailed segmentation offers insights into user preferences, helping in crafting tailor-made experiences or targeted marketing campaigns.
Regression models, deep learning networks, and ensemble methods offer a glimpse into the future. They don't just predict metrics like churn rates; they can, for instance, forecast feature adoption rates or anticipate market shifts. Businesses can then strategize proactively, staying always a step ahead.
Beyond mere outlier spotting, ML-driven anomaly detection delves deeper. With algorithms like Isolation Forest or One-Class SVM, it’s possible to identify nuanced anomalies – say, a slight but significant change in user interaction times. Such granular insights can be pivotal in preempting potential product issues.
Modern NLP techniques, powered by deep learning, can infer sentiment, context, and emotion from large volumes of unstructured user feedback. Beyond just categorizing feedback as positive or negative, ML can highlight undercurrents of user sentiment, unveiling deeper product perceptions.
Standard A/B testing can sometimes miss the forest for the trees. ML not only streamlines the testing process but also identifies compound interactions and subtle patterns, resulting in more comprehensive test outcomes. This facilitates more informed decisions on product changes.
Beyond traditional collaborative or content-based filtering, ML models can predict user needs in real-time. They adapt based on continuously evolving user behaviors, ensuring recommendations remain relevant, timely, and context-aware.
The modern business landscape is awash with data, but not all data points are of equal value or relevance. Machine learning facilitates the distillation of vast datasets into actionable insights. Instead of decisions based on surface-level interpretations, ML algorithms dive deep, discerning patterns and correlations that might escape the human eye. This allows businesses to make decisions that aren't just reactive but are rooted in a profound understanding of user behavior, preferences, and future tendencies. As a result, businesses can align their product strategy with genuine user needs and aspirations, leading to more strategic product enhancements and initiatives.
Growth, while desired, brings its own set of challenges. One of these challenges is the exponential growth of data. Traditional analytical methods can quickly become overwhelmed, leading to slower insights or even incorrect conclusions. Machine learning stands out in its ability to scale. Advanced ML algorithms, designed for high-dimensional data, can consistently process and analyze vast amounts of information without loss of speed or accuracy. This ensures that even as a business's data grows exponentially, the quality and timeliness of insights remain consistent.
In the age of digital transformation, market dynamics can change rapidly. The luxury of retrospective analysis is becoming obsolete. Real-time ML analytics continuously process incoming data, identifying shifts in user behavior, market conditions, or product performance as they happen. Businesses, equipped with these real-time insights, can pivot their strategies instantaneously, seizing emerging opportunities or mitigating emerging threats.
Generic user experiences no longer suffice in capturing and retaining user interest. Modern users expect personalization. Machine learning excels at deriving insights from each user's interactions, behaviors, and preferences. By clustering similar user behaviors or predicting individual user's next actions, businesses can craft user experiences that feel tailor-made. Such personal touches, from product recommendations to UI/UX modifications, enhance user satisfaction and loyalty.
Reactive problem-solving is often costlier and more damaging to a brand's reputation than proactive solutions. Predictive ML models, trained on historical data, can identify patterns leading to potential pitfalls or issues. Whether it's predicting server downtimes, foreseeing user churn, or identifying potential security breaches, businesses can initiate preventive measures, ensuring smooth and continuous user experiences.
Resource wastage, be it in terms of time, manpower, or capital, can be detrimental to businesses. Machine learning provides a granular analysis of high-impact areas versus low-impact ones. By discerning which features users engage with most or where user drop-offs occur, businesses can strategically allocate resources to areas that promise the highest ROI, ensuring efficiency and maximizing profitability.
Staying ahead in a competitive market requires foresight and innovation. Insights drawn from ML analytics provide businesses with a clear view of market trends, emerging user preferences, and potential industry disruptions. Armed with this knowledge, businesses can innovate, refine their product strategies, and continuously evolve to meet and exceed market expectations, ensuring they not only retain their user base but also remain market leaders.
Machine learning's introduction to product analytics is akin to equipping a craftsman with advanced tools. The base skill remains the same, but the depth, finesse, and detail of the craft improve exponentially. For businesses, this means richer insights, proactive strategies, and an ever-deepening understanding of their users.
Discover how platforms like Countly can leverage ML for deeper insights and enhanced user understanding.